A Guide to Understanding Scientific Studies (part II)
In part I of this article, we discussed common issues with scientific studies, and the benefits of open data. In this part II, we look into the different types of scientific studies and what they can (and can't) tell us.
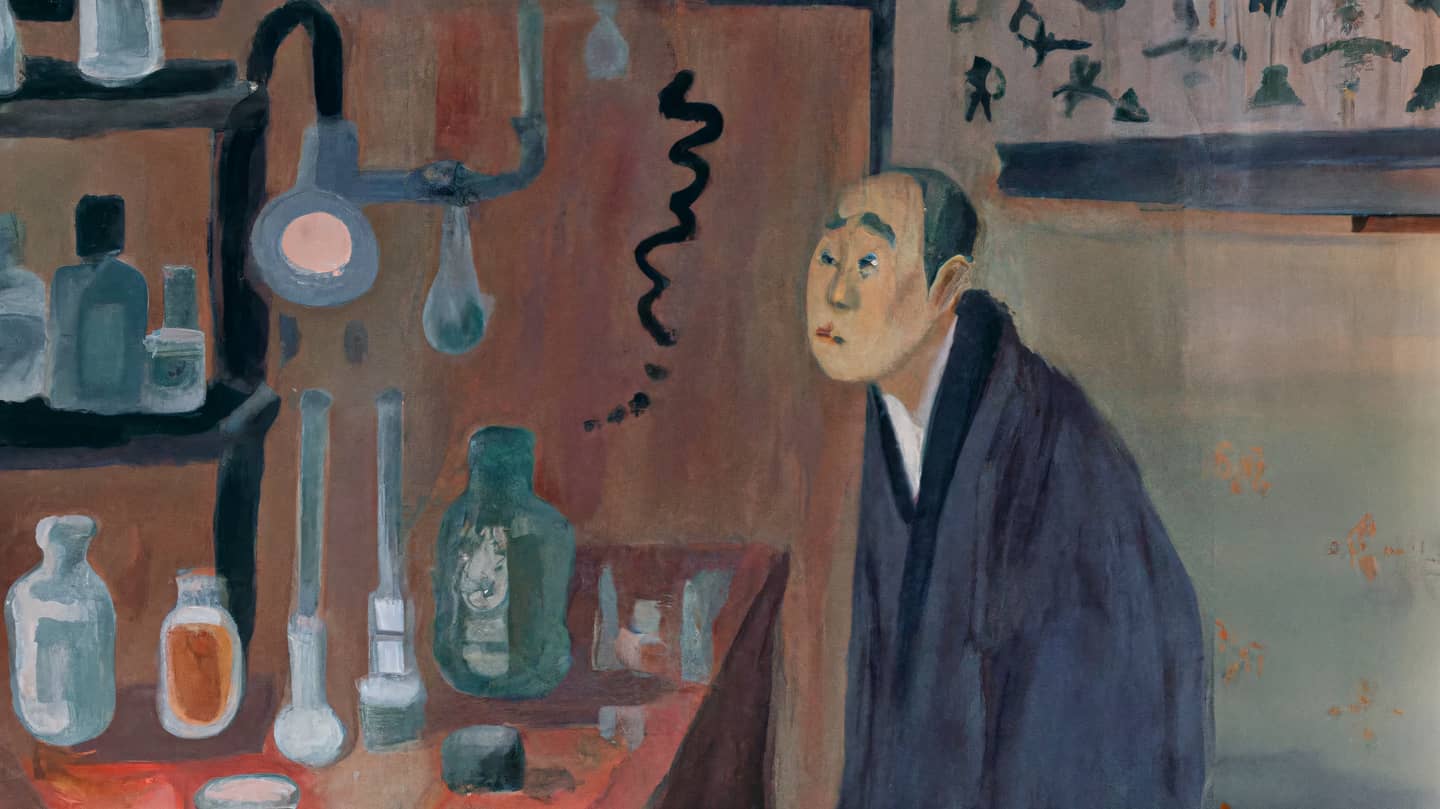
Not all Evidence is Equal
“An avocado a week can cut heart disease risk by a fifth,” The Times reported triumphantly in March 2022.
That is exciting news! So we can all just indulge in avocados and stop worrying about heart disease?
Not so fast. Let’s first examine the actual study, titled “Avocado Consumption and Risk of Cardiovascular Disease in US Adults.”
Interestingly, the title of the study is less sensational than The Times’ headline. It merely suggests a relationship between avocado consumption and cardiovascular disease (CVD) risk.
This study is classified as an “observational” study, in which the authors claim that:
[…] higher intake of avocados was associated with significantly lower risk of total CVD […]
In other words, the study finds that people who eat more avocados have a lower risk of CVD, but it doesn’t show that one causes the other.
Here, The Times has stumbled into a common pitfall: confusing an observational study (which identifies correlations, or things that happen at the same time) with an intervention study (which establishes cause and effect).
This isn’t just splitting hairs, because The Times is sending a potentially harmful message. Based on the article, people might expect that ordering an avocado salad on the side of their fried chicken and Coke will protect them against heart disease. But what if avocados aren’t especially healthy, but just a common food among people who lead overall healthier lifestyles? In that case, eating avocados won’t make any difference.
So, when we read a study, it’s crucial to understand its type and, consequently, what it can and cannot tell us.
Now, let’s delve deeper into the different types of studies.
Observational Studies
Also known as population studies, observational studies are a cost-effective way to explore and find interesting patterns in the real world. If two things consistently happen at the same time, then perhaps there is a relationship between the two that could potentially be further investigated with an intervention study.
Observational studies are also useful in situations where intervention studies are unethical or illegal.
For example, if you wanted to find out the safe level of mercury in food for a healthy individual, you wouldn’t give people different amounts of mercury and see at what dosage they fall ill. But an observational study could look at people who are already exposed to mercury (like people on diets high in fish) and look for health effects relative to mercury exposure.
Observational studies can also help disprove ideas. After all, while an observational study can’t prove that “eating avocados reduces CVD risk”, it can challenge this claim by showing that CVD risk is the same for both avocado eaters and non-eaters.
Let’s take the Hygiene Hypothesis of the 1980s as an example. This theory proposed that children who were less exposed to pathogens and parasites during early infancy would be more susceptible to allergies later.
However, a large-scale study published in the New England Journal of Medicine in 2002 found that children in the more exposed group appeared to have just as many allergies as the ones in the more sheltered group, making the Hygiene Hypothesis unlikely.
At the same time, observational studies have their limitations.
- As we’ve already seen, observational studies can’t prove cause and effect. They might find that healthy people eat more avocados, but can’t say that avocados are the reason they’re healthy.
- Observational studies often focus on relative risks which can exaggerate effects. For instance, if a study says that eating sardines doubles your risk of brain disease, that might keep you up at night. But if the absolute risk increases from 0.0001% to 0.0002%, you’ll probably not care.
- Observational studies can be thrown off by confounding factors. These are things outside the study that make it look like there’s a link when there isn’t one. For instance, in the avocado study mentioned earlier, an overall healthier lifestyle might be a confounding factor.
Types of Observational Studies
Let’s take a look at the different types observational studies.
Case Reports detail symptoms, signs, diagnosis, treatment, and follow-up of an individual patient. They often describe unique cases that can’t be explained by known diseases or syndromes. For example, a doctor might publish a case report about a patient who had a rare side effect from a common medication.
Case Series are collections of case reports involving patients who were given similar treatment. They can be useful for identifying patterns and generating hypotheses for further study. For example, early cases of COVID-19 formed a case series that helped scientists identify common symptoms and risk factors.
Cross-sectional Studies (also known as Prevalence Studies) measure the prevalence of a disease or condition in a population at a specific point in time. They are often used to find associations between risk factors and diseases. An example might be a survey of high school students to determine how many are currently smoking cigarettes.
Cohort Studies (also known as Longitudinal Studies) follow large groups of people time to see who develops a particular disease or condition. The group can be divided into those exposed and not exposed to a risk factor to see how it affects disease development. For example, the Nurses’ Health Study, which follows more than 100,000 nurses and has produced many insights into risk factors for major chronic diseases in women.
Case-Control Studies start with people who have a disease (cases) and compare them to people who do not have the disease (controls), looking backwards in time to assess their exposure to a potential risk factor. These studies are often used when studying rare diseases or diseases with a long latency period (like cancer). An example would be a study looking at lung cancer patients and non-cancer patients and their past smoking habits.
Intervention Studies
An intervention study is an experiment in which researchers change something, then measure the effect of that change, while trying to keep other factors as much the same as possible.
Participants in these studies might undergo a novel medical treatment, begin a new exercise program, alter their diet, or participate in psychological therapy.
Intervention studies generally come in a few different types, each with different goals and uses:
Randomized Controlled Trials (RCTs)
RCTs are commonly used to test new drugs, medical procedures, or other therapeutic interventions. Regarded as the gold standard of intervention studies, RCTs excel in establishing cause and effect.
In an RCT, participants are randomly assigned to:
- an intervention group that receives the treatment, and
- a control group that doesn’t.
This ensures that any differences observed between the two groups can be attributed to the intervention, instead of some outside factor.
There are different types of RCTs, depending on how they’re carried out:
-
Single-blind trials: The participants don’t know whether they are in the intervention group or the control group, but the researchers do. This helps to prevent bias in the participants’ responses.
-
Double-blind trials: Both the participants and the researchers don’t know who is in the intervention group or the control group. This helps to prevent bias in the participants’ responses and the researchers’ interpretation of the data.
-
Triple-blind trials: In addition to the participants and researchers, the statisticians analyzing the trial data also don’t know who is in which group. This provides another layer of bias protection.
The ‘blinding’ helps minimize bias, giving us more confidence in the results. But conducting these studies can be challenging, and they may not always be feasible. Nevertheless, well-conducted RCTs provide the highest level of evidence about the effectiveness of a treatment or intervention.
So, when you see a study described as a ‘randomized, triple-blind, placebo-controlled trial’, you know it’s serious business.
Non-Randomized Controlled Trials
Sometimes, researchers can’t randomly decide who gets a certain treatment or who doesn’t. This is where non-randomized controlled trials come in.
For example, imagine a study on a new exercise program for stroke recovery. Let’s say patients who arrive at the hospital earlier get to start the program sooner (the treatment group). Those who arrive later start the program at a later time (the control group). This isn’t random - it’s based on when the patients get to the hospital. So, while this study can still provide useful information, we have to be a bit more cautious with the results because other factors (like how quickly a patient got to the hospital) might affect the outcome.
Before-and-After Studies
In these studies, researchers measure something (like students’ test scores), apply a change (like a new teaching method), and then measure the same thing again. This helps them understand if the change made a difference.
A downside of this method is that an observed difference is not necessarily caused by the change.
Cross-Over Studies
In these studies, each participant gets to try both the new thing being tested (like a medicine) and a control (like a placebo pill). They try these at different times, and researchers compare how each one worked. This helps when the effect of the intervention is temporary and can be reversed.
For example: To test a new pain medicine, each participant would take the new medicine and a placebo at different times. Researchers then compare how much each participant’s pain changed under each condition.
Community Trials
These studies involve entire groups or communities, not just individuals. Researchers give different groups different things (like different school programs) and see how the whole group changes.
For example: In a study to test a new school lunch program, different schools get different lunch menus. The researchers then compare the health of the students at each school.
Quasi-Experimental Studies
In these studies, researchers can’t randomly assign participants to groups. Instead, they select groups based on specific criteria.
For example: To see if a new teaching method helps students graduate, researchers might compare schools that are using the new method to similar schools that are not.
A downside of this method is that if there are more graduates from the schools using the new method, it might be because of the new teaching method, but also because of some other reason.
Meta-Analyses
Imagine you have a group of studies, all asking similar questions. Some of the studies might have a small sample size, while others are larger. Some found strong effects, while others found weak or no effects. How do you make sense of all this?
A meta-analysis is a mathematical approach to combine the results from multiple studies to arrive at a pooled estimate of effect.
However, if the included studies are biased, of poor quality, or very different from each other, the results could actually be misleading. A meta-analysis is never better than the studies that it is based on.
Systematic Reviews
While a meta-analysis combines the results of multiple studies into a single statistical estimate, a systematic review takes a broader approach.
Systematic reviews aim to bring together all the empirical evidence on a given topic, to create a comprehensive, unbiased overview.
Systematic reviews often include a meta-analysis, but not always. If the studies are too different from each other to meaningfully combine their results, the systematic review might just summarize the findings.
As with meta-analyses, the quality of a systematic review depends on the quality of the included studies. And the rigor with which it’s carried out: Did the researchers find all relevant studies? Did they assess the quality of the studies? Did they account for bias?
Narrative Reviews
Narrative reviews are broad overviews of a topic, written by experts. They usually don’t use a specific method to search for or analyze studies.
These reviews give insights and opinions, but they are not as strong as evidence compared to systematic reviews or meta-analyses. This is because they can be more opinion-based and less systematic.
Other Types of Studies
There’s other papers out there still. Some summarize what we currently know on a topic, while others offer perspectives, commentary, or opinions on existing research. While these don’t give us new data, they help us make sense of the data we have.
To be Continued
In the next episode, we’re going to talk about how to apply all this in practice!