Beware the Man in the White Coat: A Guide to Understanding Scientific Studies (part III)
In this final episode of our guide to reading scientific studies, a practical step-by-step approach to help you tell the good from the bad.
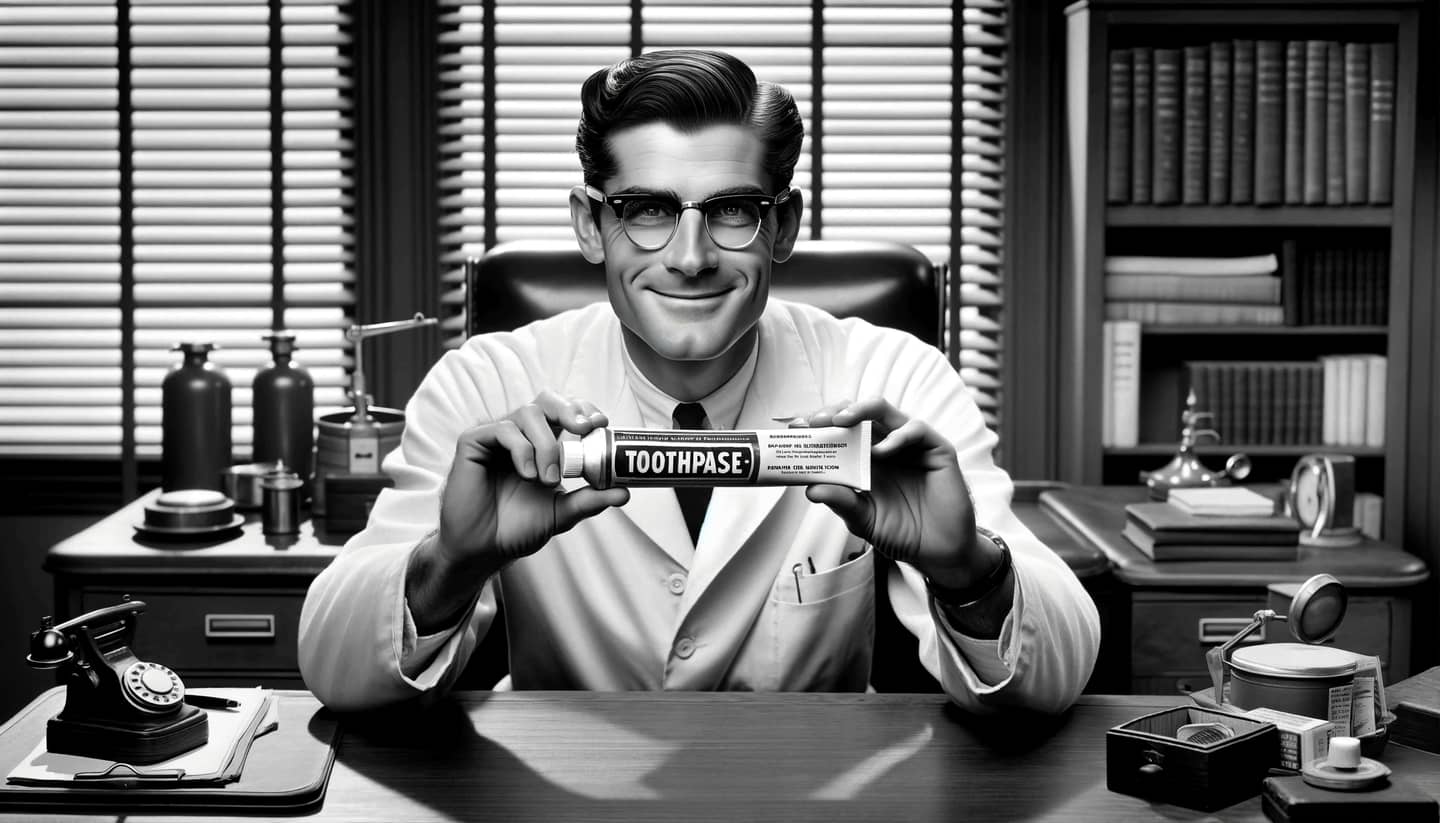
Beware the Man in the White Coat
In articles, presentations, and everyday discussions, science is often used to add weight to arguments. People say things like “studies prove…” or “research has shown…” Whenever I hear those phrases, I think about the Man in the White Coat.
You might remember him from old TV commercials, endorsing toothpaste, washing powder, and yes, even cigarettes. Though he’s no longer on TV, he still pops up in conversations and presentations, trying to persuade us into buying his product or his argument.
Question is, should we believe him?
A Telephone Game
The answer is: not without due diligence. Here’s why.
Scientific claims transform as they’re passed along, much like in a Telephone Game:
- The speaker may be cherrypicking from a mainstream or professional publication, influenced by confirmation bias.
- The publication itself may misquote a scientific study to make a good headline. Remember from part II how The Times turned “healthy people eat more avocados” into “eating avocados makes you healthy”?
But even if a scientific claim is quoted correctly, it is likely to be incorrect or overstated to begin with, as we discussed in part I.
Based on these odds, it is rational to approach a claim with a healthy dose of skepticism.
So, what do we do?
This is all well and good, you might say, but then should we dismiss any quoted scientific claim outright? Surely some of those must be true. But how would we know? Let’s find out.
1. Go back to the source.
Whenever someone quotes a scientific claim, the first step is to check with the original source.
This can be more fun than you might think. You’ll notice that when you ask a speaker to provide the source of their claim, they often won’t recall, or promise to “get back to you,” only to go silent. Needless to say, you can then safely dismiss their claim.
If they do share a link to the study, you’ll often find that the quoted information doesn’t align with what the study actually says.
If the study does align with the speaker’s claim, it may still well be false, checking it will take a bit more work.
2. Check the study’s timeline.
In part I, we covered HARKing, or Hypothesizing After Results are Known. It’s a form of cheating where researchers change their study design after seeing the results to make them appear more favorable.
If a study’s design has been altered to align with its results, the evidence is likely to be misleading, if not entirely false.
How do we uncover HARKing? By examining the study’s timeline.
Specifically, you should compare the registration date of the study design with the start date of the research.
- If the study design was registered before the actual research began, we don’t need to worry about HARKing.
- If the registration date is after the research start date however, or if the study has no timeline at all, HARKing can’t be ruled out. This doesn’t invalidate the study outright, but we need to approach it cautiously.
Is the study passing this test? That’s great! Unfortunately, most scientific studies don’t pass the next test. So let’s move on and see what we find.
3. Look for raw data and statistical methods.
Raw data and statistical methods are essential because without those, a study cannot be reproduced, and boils down to “trust me science”. As product managers we say: trust, but verify! If a study cannot be independently replicated, it has no credibility.
You’ll need to look for download links to the study’s complete raw data and statistical methods. If they are present, that’s excellent! You can now have some trust in the study’s findings.
More likely however, you won’t find any links, which means we can’t have confidence in the study’s claims.
4. Identify intervention studies.
Most studies you will find in the media fall under the category of population studies (also known as observational studies), which tend to provide weak or misleading outcomes.
They essentially say, “Here are two things happening at the same time; there might be a connection, but further investigation is needed.” Such outcomes are valuable mainly for academics looking for topics for research, but not for the rest of us.
Again, observational studies include:
- case reports
- case series
- cross-sectional studies
- cohort studies
- case-control studies
So when you encounter any of these, think “not for me”.
Meta-analyses and systematic reviews are problematic too. Their quality varies widely, and only experienced researchers can distinguish between good and bad ones. For reluctant product managers like us, they aren’t worth our time.
However if you find an intervention study (such as a clinical trial, case study, or cross-over study) with links to full raw data and statistical methods, that’s a different story. The chances of finding valuable evidence are much higher, and we can approach these studies with an open mind and cautious optimism.
As discussed earlier, the most rigorous intervention studies are triple-blind randomized controlled trials. When participants, researchers, and statisticians conduct the study without knowing who is in the intervention group and who in the control group, there is less risk of bias.
5. Understand the Strength of the Evidence
Even when we come across a triple-blind randomized controlled trial that was pre-registered and has links to raw data and statistical methods, the results may still not be meaningful.
Whereas it takes domain knowledge and statistical skills to fully understand the results, there are some simpler indicators that you can use to get an idea of their significance.
- Participant Count: In a randomised clinical trial, the number of participants should be large enough to even out random differences. There’s no hard number, as it depends on what’s being measured. What we can say is that given two studies with the same study design, the one with the higher participant count should yield more reliable results.
- Study Duration: The study duration needs to align with the research goals. For example, in a cross-over study evaluating the effect of solo versus pair programming, developers need time to adapt when transitioning between the two. Meanwhile, in a nutrition study about diabetes risk, a much longer study duration is necessary.
- Surrogate Endpoints: Let’s say you’re researching the effect of sugar intake on the risk of heart failure. A full-blown study on this would be long, costly, and ethically problematic – you can’t just give people lots of sugar and see if it harms them. So instead, researchers might look at easier-to-measure markers like blood pressure or long-term blood sugar levels. These are called surrogate endpoints and they are much less reliable than actual ones.
- Probability (p-value): Probability is a measure in statistics that helps determine the significance of results. Generally, p-values below 0.05 are considered significant, above 0.05, weak. The value of 0.05 is arbitrary, and as you’ll remember from part I, p-values can be manipulated. So unless you have access to the raw data, don’t take them at face value.
Does Peer-Review Matter?
You’ve probably heard about “peer-reviewed” studies and how they’re considered the gold standard in science. But here’s a reality check: they aren’t.
Peer-review is an expert’s check on a scientific paper before it’s published.
The problem is that we have zero insight into the process. We don’t know who the experts are, or how they reviewed the study. (Any chance that a reviewer who rejects too many studies won’t be asked by the publisher again?)
Another problem is that peer-reviewers need to be, by definition, experts in the same field as the researchers of the study. That means they are not necessarly unbiased and might even have an interest in the study to fail.
The Reproducibility Project led by Brian Nosek, that we mentioned in Part I of this series, revealed that the majority of the studies that they examined was not reproducible. These studies were all peer-reviewed and published in major publications.
In other words, peer-review does an atrocious job of what it is supposed to do: filtering out bad science. We must dismiss it as a badge of quality.
Bringing it all Together
This is all a lot of information, you might say. Isn’t there a simpler way to gauge a study’s credibility?
Fortunately, there are three clear indicators that you can check with relative ease. By awarding points to these indicators, you get a confidence score:
- +1 Point: If the study design was registered in advance, proving that no HARKing took place.
- +2 Points: If the study directly links to the full raw research data, so that it can be verified.
- +2 Points: If the study, in addition, directly links to the statistical methods, making the outcomes reproducible.
From 3 points onwards, we can have some confidence in the results, and of course, more points is better.
The point system only applies to randomised controlled trials (RCTs). Other types of studies get 0 points — not because they are all bad, but because as reluctant product managers, we have the skills nor the time to interpret them properly.
Conclusion
You should now be better equipped to read, interpret, and use scientific studies to your advantage.
Thanks for reading and let me know if you have any feedback!